Title: “Building and understanding brains: How can AI research inform neuroscience? ”
Date: Saturday 9 July 2022, 15:15-16:45
The “Brain Debate” is a panel discussion on the topic of “Building and understanding brains: how can AI research inform neuroscience?”
Panelists will discuss how new work in AI research can guide our understanding of biological brains, across levels of description, from cells to systems to behaviour. For example, do humans learn like machine learning models, by gradient descent? Is there a biological analogue for backpropagation? Are biological systems best described as learning from supervision, by reinforcement, or in an unsupervised fashion? How can we build artificial systems that are robust and sample-efficient, like biological systems? What is the outlook for neuroscience in the new age of AI research? There will be short (2-3 slide) presentations on this theme, questions from the audience, and discussion among the panel.
Speakers and discussants:

Claudia Clopath
Imperial College LondonProfessor Claudia Clopath is based in the Bioengineering Department at Imperial College London. She is heading the Computational Neuroscience Laboratory.
Her research interests are in the field of neuroscience, especially insofar as it addresses the questions of learning and memory. She uses mathematical and computational tools to model synaptic plasticity, and to study its functional implications in artificial neural networks.
Prof. Clopath holds an MSc in Physics from the EPFL and did her PhD in Computer Science under Wulfram Gerstner. Before joining Imperial College, she did postdoctoral fellowships in neuroscience with Nicolas Brunel at Paris Descartes and in the Center for Theoretical Neuroscience at Columbia University. She published highly cited articles in top journals such as Science and Nature, has given dozens of invited talks and keynotes around the world, and received various prizes such as the Google Faculty Award in 2015.
Claudia Clopath
Imperial College London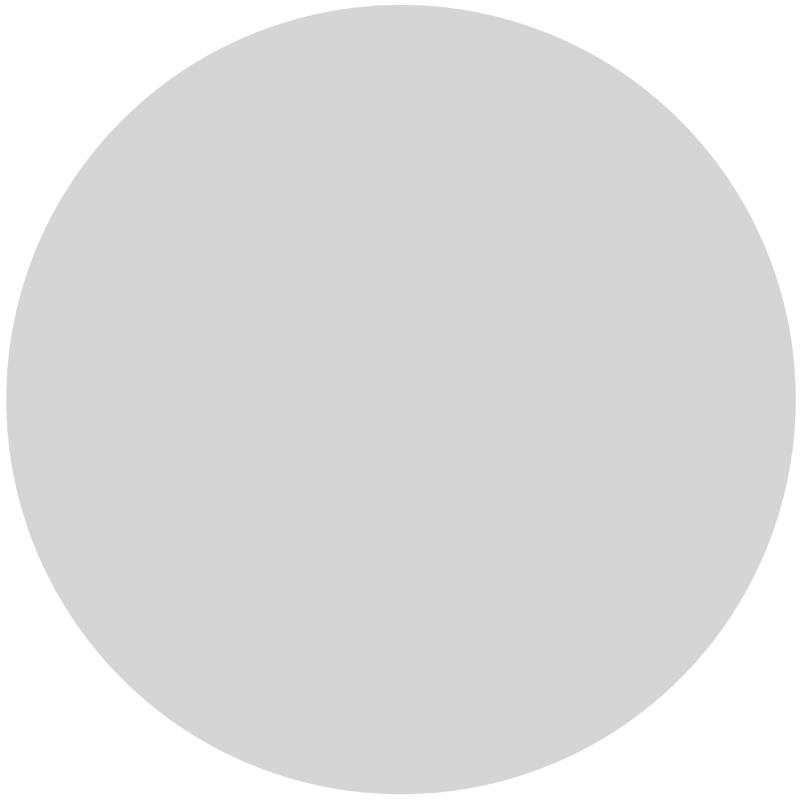
Stanislas Dehaene
College de FranceStanislas Dehaene
College de France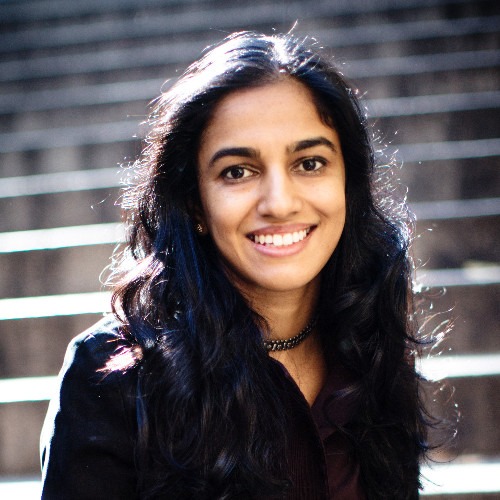
Kanaka Rajan
Icahn School of Medicine at Mount SinaiKanaka Rajan, Ph.D. is a Computational Neuroscientist and Assistant Professor at the Friedman Brain Institute at the Icahn School of Medicine at Mount Sinai in New York. Her research seeks to understand how important cognitive functions — such as learning, remembering, and deciding — emerge from the cooperative activity of multi-scale neural processes. Using data from neuroscience experiments, Kanaka applies computational frameworks derived from machine learning and statistical physics to uncover integrative theories about the brain that bridge neurobiology and artificial intelligence.
Prior to joining the faculty at Mount Sinai, Kanaka completed her postdoctoral training at Princeton University and her doctoral work at Columbia University, where she made significant contributions to understanding feature selectivity and recurrent neural networks (RNNs) by pioneering computational approaches for understanding complex brain processes.
Dr. Rajan’s work has been recognized with several awards (Allen Institute’s Next Generation Leaders Council, The Harold and Golden Lamport Basic Science Research Award, Young Investigator Award from the Brain and Behavior Foundation, Understanding Human Cognition Scholar Award from the James S McDonnell Foundation, Sloan Research Fellowship), and her work is supported by the NIH BRAIN Initiative and the NSF. For more information about Dr. Rajan, please visit www.rajanlab.com.
Kanaka Rajan
Icahn School of Medicine at Mount Sinai
Josh Tenenbaum
MITJosh Tenenbaum is Professor of Computational Cognitive Science at the Massachusetts Institute of Technology in the Department of Brain and Cognitive Sciences, the Computer Science and Artificial Intelligence Laboratory (CSAIL) and the Center for Brains, Minds and Machines (CBMM). He received a BS from Yale University (1993) and a PhD from MIT (1999). His long-term goal is to reverse-engineer intelligence in the human mind and brain, and use these insights to engineer more human-like machine intelligence. In cognitive science, he is best known for developing theories of cognition as probabilistic inference in structured generative models, and applications to concept learning, causal reasoning, language acquisition, visual perception, intuitive physics, and theory of mind. In AI, he and his group have developed widely influential models for nonlinear dimensionality reduction, probabilistic programming, and Bayesian unsupervised learning and structure discovery. His current research focuses on the development of common sense in children and machines, the neural basis of common sense, and models of learning as program synthesis. His work has been recognized with awards at conferences in Cognitive Science, Philosophy and Psychology, Computer Vision, Neural Information Processing Systems, Reinforcement Learning and Decision Making, and Robotics. He is the recipient of the Troland Research Award from the National Academy of Sciences (2012), the Howard Crosby Warren Medal from the Society of Experimental Psychologists (2015), the R&D Magazine Innovator of the Year (2018), and a MacArthur Fellowship (2019), and he is an elected member of the American Academy of Arts and Sciences.
Josh Tenenbaum
MIT
Jane Wang
Staff Research Scientist, Neurosciencewww.janexwang.com
Jane is a staff research scientist at DeepMind, where, as a member of the neuroscience team, she works to create and understand novel approaches for learning and meta-learning in a reinforcement learning context, inspired by the latest advancements in cognitive neuroscience. She obtained her Ph.D in Applied Physics from the University of Michigan, where she studied complex systems, physics, and computational neuroscience, before moving on to conduct research in cognitive neuroscience at Northwestern University. She also enjoys science fiction and aerial arts.
Jane Wang
Staff Research Scientist, NeuroscienceModerator:

Christopher Summerfield
OxfordChristopher Summerfield is a Professor in the Department of Experimental Psychology at the University of Oxford. He is also a research scientist at Deepmind. His work focusses on how humans learn and make decisions, using behaviour, imaging, and computational modelling techniques.